What Are Guardrails
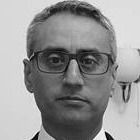
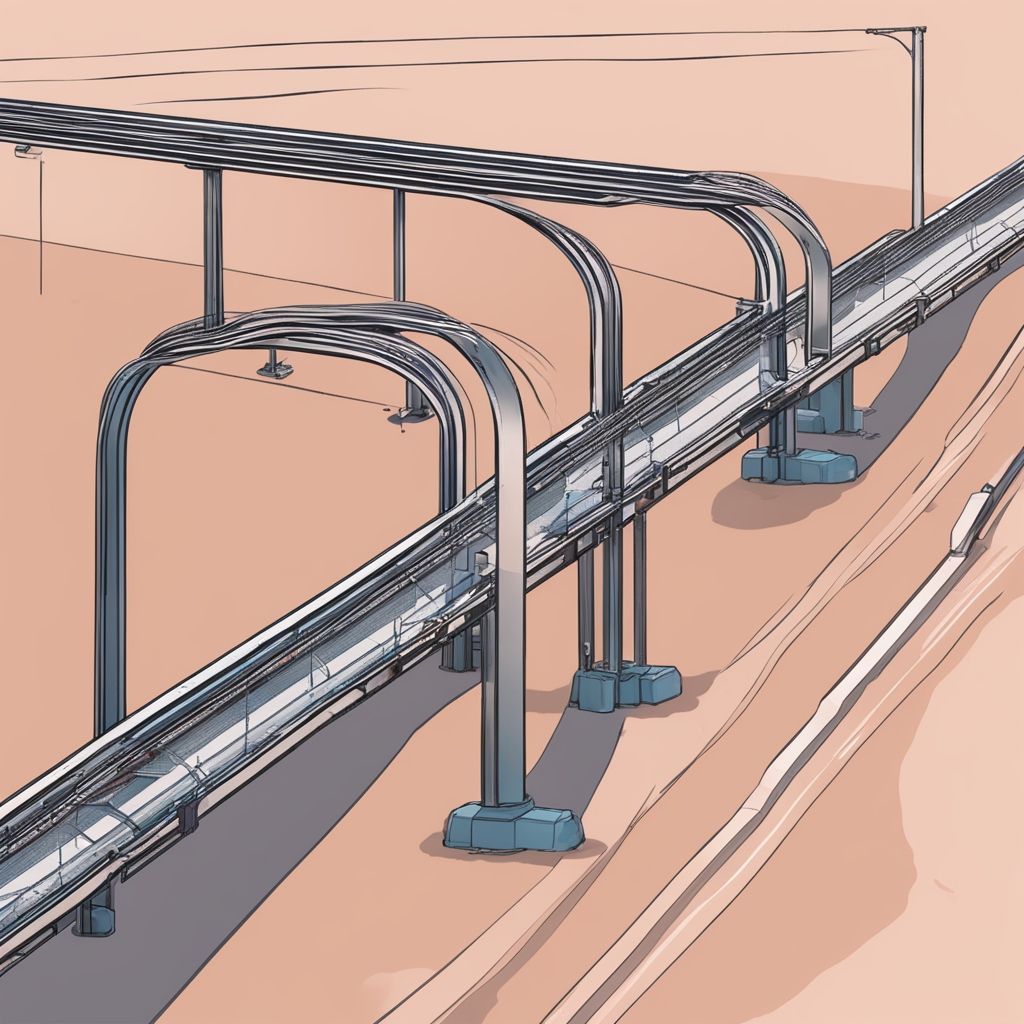
As the world increasingly adopts large language models (LLMs) for various applications, one critical concept has become central to their responsible use—guardrails. Guardrails are predefined restrictions or guidelines that ensure AI systems behave safely, ethically, and align with their intended purposes. These mechanisms prevent the models from generating harmful, inappropriate, or undesirable content, especially when used in sensitive environments like healthcare and education.
Guardrails can range from restricting the output of hate speech, misinformation, or unethical recommendations to limiting the interaction between AI and end-users in a controlled, secure manner. They are not merely a safety feature but an essential tool that enables developers and organisations to maintain control over AI systems while ensuring positive user experiences.
Guardrails Are Already in Most LLMs
Most large language models today already come with built-in guardrails designed by their creators. These pre-integrated mechanisms are meant to filter out inappropriate content and steer the model's behaviour toward acceptable standards. For instance, LLMs typically include mechanisms that:
- Content Filtering: Automatically detect and block harmful or inappropriate language, such as profanity, hate speech, or graphic content.
- Ethical Constraints: Prevent the model from generating responses that violate ethical norms, such as promoting violence, discrimination, or unsafe behaviours.
- Policy Enforcement: Ensure that the AI operates within predefined boundaries, such as respecting user privacy or complying with regulatory requirements in various industries.
These guardrails are often an intrinsic part of popular LLMs, ensuring that developers and end-users don't inadvertently deploy models that could cause harm. In some cases, these safety mechanisms may also be customizable, allowing organisations to adapt the restrictions to their specific requirements.
Outside of the base model sphere guardrails can also be implemented through a combination of techniques, including prompt engineering, response filtering, fine-tuning, and continuous monitoring.
Adding Your Own Guardrails
While built-in guardrails are crucial, they may not fully cover specific use cases. For more control, you can add custom guardrails to an LLM through various methods. Here are some approaches:
- Prompt Engineering: Crafting prompts in a way that limits the range of responses. For example, you can instruct the model to avoid generating speculative content or to stick to factual statements.
- Post-Processing Filters: Analysing the output of the LLM after generation and applying filters to remove undesirable responses. This can be done using additional machine learning models, regex rules, or keyword detection.
- Fine-Tuning: Fine-tuning the model on a dataset that reflects your desired behaviour can further refine and reinforce the behaviour of the LLM.
- Contextual Constraints: Implementing dynamic constraints based on user interaction context. For instance, limiting the number of questions the user can ask in a sensitive domain, such as medical advice.
Categories of Guardrails
To effectively manage the behaviour of LLMs, we have categorised guardrails into five key areas: Security & Privacy, Response Relevance, Language Quality, Content Validation & Integrity, and Logic & Functionality Verification. For each category, we offer examples of the types of guardrails that can be implemented.
1. Security & Privacy
- Inappropriate Content Filter : One of the most critical guardrails, this filter prevents the generation of explicit, offensive, or harmful content, such as graphic descriptions or NSFW material.
- Offensive Language Filter: This guardrail actively detects and removes profane or disrespectful language, ensuring that the LLM communicates in a civil and respectful tone, suitable for all audiences.
- Prompt Injection Shield: This advanced guardrail protects the LLM from prompt injection attacks, where malicious users attempt to manipulate the system to generate unintended responses by crafting deceptive inputs.
- Sensitive Content Scanner: This tool identifies and flags sensitive information, like personal data or potentially harmful content, ensuring privacy and preventing the unintentional disclosure of confidential information.
2. Responses & Relevance
- Relevance Validator: This guardrail ensures that the LLM’s responses are contextually aligned with the user’s query. It prevents the model from providing answers that may be accurate but irrelevant to the user's intent.
- Prompt Address Confirmation: This tool ensures that the model remains focused on the user's query and doesn't drift into unrelated topics or provide ambiguous answers.
- URL Availability Validator: When the LLM generates URLs or links, this validator checks whether the links are active and functional, reducing user frustration from broken or inactive links.
- Fact-Check Validator: This is one of the most crucial guardrails for ensuring accuracy. It verifies the information generated by the LLM to ensure that no misinformation is propagated, especially in domains requiring high factual accuracy, like healthcare, legal, or financial services.
3. Language Quality
-
Response Quality Grader: This tool evaluates the quality of the generated text, assessing clarity, relevance, and logical structure. It ensures that the LLM produces not only accurate but also well-written and easily understandable responses.
-
Duplicate Sentence Eliminator: To enhance conciseness, this filter removes any redundant or repetitive sentences from the LLM’s responses, improving readability.
4. Content Validation and Integrity
- Competitor Mention Blocker: In specific commercial applications, this guardrail prevents the LLM from mentioning or promoting competitors, ensuring that content remains focused on the desired brands or topics.
- Price Quote Validator: This tool checks any price quotes generated by the LLM for accuracy and validity, particularly in e-commerce or business applications, preventing customer disputes due to incorrect pricing.
- Source Context Verifier: When the model references external content or sources, this guardrail ensures that the information aligns accurately with the original source, avoiding misrepresentation or out-of-context quoting.
- Gibberish Content Filter: This guardrail ensures that the LLM doesn’t produce incoherent or nonsensical responses by filter
5. Logic and Functionality Validation
- SQL Query Validator: For use cases where the LLM generates database queries, this tool ensures that SQL queries are valid, safe, and functional, minimising errors and security risks.
- JSON Format Validator: When LLMs output JSON structures, this tool ensures that the generated format adheres to correct syntax, avoiding issues in downstream applications.
- Logical Consistency Checker: LLMs may occasionally generate content that is internally contradictory or illogical. This checker identifies such inconsistencies and ensures the output remains coherent and sensible.
Conclusion
Guardrails are essential to the responsible deployment of large language models. By understanding the built-in mechanisms and implementing your own, you can ensure the safe, ethical, and effective use of AI systems across various applications. Whether you are aiming to prevent the spread of misinformation, enforce company policies, or ensure a positive user experience, guardrails are a powerful tool for shaping how LLMs interact with the world.